Why Data Analysis Is Not Just For Big Companies
Contrary to what you may think, data is not a complicated concept that you need to master for five years before you can even be considered for a job. Most businesses collect data in some form or another, but don’t treat it as an asset until they try to grow – and depending on how that data collection happens, by that point it may be incomplete, irrelevant, or unsuitable for purpose.
Before you log out, put off by the thought of stats and maths, can you answer this: Did you check the weather before you left the house today?
Or maybe you scrolled through the newspaper on your commute to work?
How about walking by your favourite restaurant to see if they have any lunchtime specials today?
Those are all examples of people accessing and using data.
Chances are, you used data half a dozen times before you even switched on your computer this morning. Analysing data is just being systemic about it – and you don’t need much to develop the skill.
So what is data analysis, really?
To over-simplify, data is what information is derived from. It is the building blocks upon which major decision-making is (or should be) built.
Data helps us make informed decisions
Data on weather tells us whether it is likely to rain, which helps us decide whether to take an umbrella. Data from a canteen kitchen tells us what is on the menu, which then helps us decide whether to buy a sandwich or take our chances with the ‘surprise’ special of the day.
So far, so good. But most decision-making isn’t straightforward, and even the way that data is interpreted is not always helpful. Just because it’s cloudy outside, it doesn’t mean it’s going to rain.
Data analysis is the systematic review of data, in order to get appropriate information from it. Depending on how rigorous the analysis was – how closely the rules were followed, and what the external factors were – the resulting information is given due weight in the decision-making.
To put it in a different way, if you based your decision not to take an umbrella on little more than gut feeling, that’s not the result of rigorous data analysis. But if you took the time to look at information from several sites with a proven track record, you have probably done ‘due diligence’ on how likely you are to get caught up in a storm.
How can data analysis be learned?
Most business decisions are a lot more complicated than the example just given. If one misjudges the likelihood of rain, the consequences are a cold and a ruined coat. If one misjudges the market for their business, they will, at a minimum, lose a fair amount of money and time. So it’s not surprising that business owners want the best possible information, and the analysis to be carried out appropriately.
Does that mean that the only data analysts worth listening to are the ones with a PhD? No.
Certainly, there are companies and positions in which high degrees of statistical analysis and experience are needed. But if you’re a small business, the chances are you can get started quickly, and with minimal fuss – and you can train your junior employees to apply the same principles to their work as well.
Your Key Principles for Data Analysis
Data analysis needs to be systematic, self-critical, and clear about its goals. Where the data is not sufficient to make a conclusion, you must be ready to go back to the source (or the customer survey) in order to find out more.
For an analysis to be systematic, it should follow an established process of steps, so that resulting information can be traced back and that the process can be repeated again.
Let’s imagine you own a store. You want to identify your bestselling item of the month. The process to do so would look something like this:
Step 1: Get all sales receipts.
Step 2: Note down the data, such as type of product, price, and when it was sold.
Step 3: Go over that data to find the product that is sold the most.
Step 4: Repeat process the next month.
Depending on how deep you want to go, your process can include steps 3.1, 3.2… 3.15 to figure out which days you sold the most products, whether certain products were often sold together, what are the peak times during the day, and whether sales and promotions had any significant impact on the sale of a specific product or not. Doing this on a regular basis means you have up to date information that can be used to make decisions, negotiations etc. If in doubt, you can go back and check the steps, and make amends to your process if you find that certain steps are becoming difficult (like switching from paper-based calculations to Excel, for example, or using e-receipts).
Self-critical analysis is one that asks uncomfortable questions of itself
That may seem daunting, but in practical terms, this boils down to asking oneself:
What is the data?
Where does it come from?
What is missing?
Am I interpreting it correctly?
What are my limitations?
In the context of our example, the data in question is from store receipts. It comes from the register and/or online sales system. Plenty of things can still be missing from it – whether the person was buying an item as a gift or for themselves, how far they travelled from, whether they are a repeat customer or not, and whether the item was returned later. In some cases, a store owner might decide that this is important data to have, so they build into their system a step to record such things (feedback sheets, customer surveys, recording reasons for return) but it isn’t information that can be derived from a receipt by itself.
Whether or not an interpretation is correct is likely the most self-critical question that can be asked, and it is useful if a business ever anticipates their information and decision-making being challenged. If you removed a beloved item from your store because of low sales, and someone objected, you can go back to the data. Perhaps the sales really were low – or, perhaps, they were only low during a particular time of the year, after which they picked up again. It’s okay to have alternative explanations for what you see in the data – and there are statistical tests you can run to check off some of them – but the main thing to remember is that you consider all options in your business’ context.
The sales of the beloved item may spike in certain periods, but if 80% gets returned within two weeks, then you know the decision to discontinue it was as good one.
The clarity of goals
This is the final principle and one that needs to run through the other two, as well as the data collection. It’s okay not to be sure about your goals – this is why taking in what the data is, where it comes from, and what is missing is important. If you decide that you simply don’t have enough data to get the information you’re looking for, it may be time to get back to that survey or that returns process and figure out if there is a way to record the data that is missing. What does not help is collecting data for the sake of it, without a specific use in mind.
It’s one thing to just record expenditure and profits for the sake of taxes. It’s another to go carefully through all receipts in order to figure out your shop’s strengths and weaknesses. If you’re not thinking about using that data strategically, why waste time and effort recording it?
Obviously, data analysis will look different for every business. But the main point is this: just because it is difficult does not make it impossible. What’s important is being willing to go out on a limb and adapt as you go along.
Related Posts
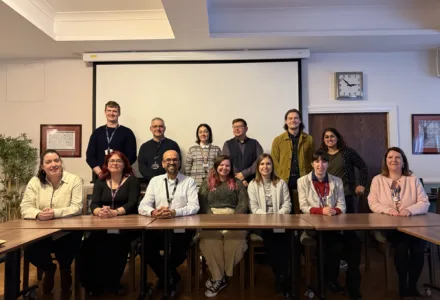
Visits to BGU from Innovate UK
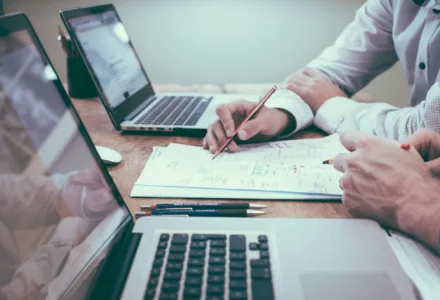
LORIC Wraps Up Successful SED Project
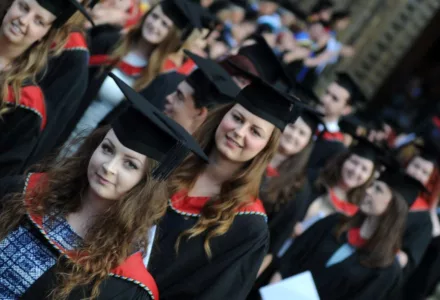
Innovate UK’s East Midlands Knowledge Exchange Forum hosted on BGU campus!
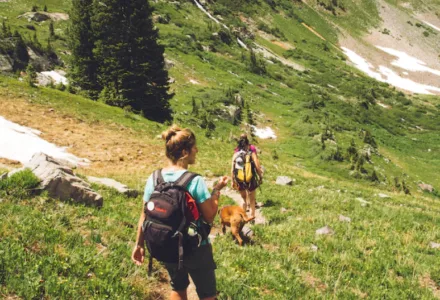
Understanding the Economic Impact and Future Potential of Greater Lincolnshire’s Sport, Physical Activity & Leisure Sector
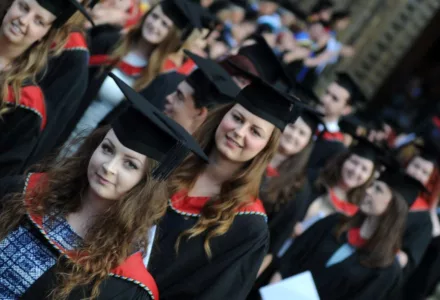
LORIC Wins Venue of the Year Award
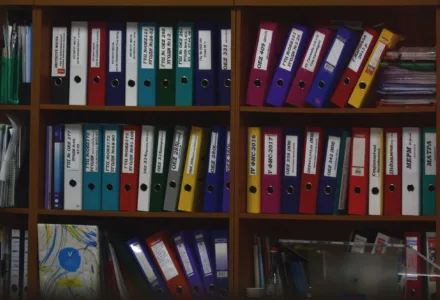
Digitising the Archive of the 20th Century Philosopher John Macmurray